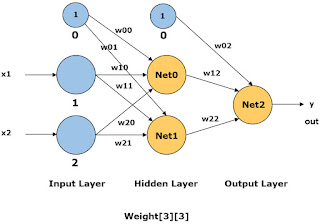
Machine Learning, Tom Mitchell, McGraw Hill, 1997.
http://www.cs.cmu.edu/~tom/mlbook.html
_______________________________________________________________
#define BP_LEARNING(float)(0.5)// The learning coefficient.
class CBPNet{
public:
CBPNet();
~CBPNet() {};
float Train(float, float, float);
float Run(float, float);
private:
float m_fWeights[3][3];// Weights for the 3 neurons.
float Sigmoid(float);// The sigmoid function.
};
CBPNet::CBPNet()
{
srand((unsigned)(time(NULL)));
for (inti=0;i<3;i++) {
for (intj=0;j<3;j++) {
m_fWeights[i][j] = (float)(rand())/(32767/2) -1;
}
}
}
float CBPNet::Train(floati1, float i2, float y) {
float net1, net2, i3, i4, out;
// Calculate the net values for the hidden layer neurons.
net1 = 1 * m_fWeights[0][0] + i1 * m_fWeights[1][0] + i2 * m_fWeights[2][0];
net2 = 1 * m_fWeights[0][1] + i1 * m_fWeights[1][1] + i2 * m_fWeights[2][1];
// Use the activation function-the Sigmoid.
i3 = Sigmoid(net1);
i4 = Sigmoid(net2);
// Now, calculate the net for the final output layer.
net1 = 1 * m_fWeights[0][2] + i3 * m_fWeights[1][2] + i4 * m_fWeights[2][2];
out = Sigmoid(net1);
// We have to calculate the errors backwards from the output layer to the hidden layer
float deltas[3];
deltas[2] = out*(1-out)*(y-out);
deltas[1] = i4*(1-i4)*(m_fWeights[2][2])*(deltas[2]);
deltas[0] = i3*(1-i3)*(m_fWeights[1][2])*(deltas[2]);
// Now, alter the weights accordingly.
float v1 = i1, v2 = i2;
for(int i=0;i<3;i++) {
// Change the values for the output layer, if necessary.
if (i == 2) {
v1 = i3;
v2 = i4;
}
m_fWeights[0][i] += BP_LEARNING*1*deltas[i];
m_fWeights[1][i] += BP_LEARNING*v1*deltas[i];
m_fWeights[2][i] += BP_LEARNING*v2*deltas[i];
}
return out;
}
float CBPNet::Sigmoid(floatnum) {
return (float)(1/(1+exp(-num)));
}
float CBPNet::Run(floati1, float i2) {
float net1, net2, i3, i4;
net1 = 1 * m_fWeights[0][0] + i1 * m_fWeights[1][0] + i2 * m_fWeights[2][0];
net2 = 1 * m_fWeights[0][1] + i1 * m_fWeights[1][1] + i2 * m_fWeights[2][1];
i3 = Sigmoid(net1);
i4 = Sigmoid(net2);
net1 = 1 * m_fWeights[0][2] + i3 * m_fWeights[1][2] + i4 * m_fWeights[2][2];
return Sigmoid(net1);
}
#define BPM_ITER2000
void main() {
CBPNetbp;
for (inti=0;i<BPM_ITER;i++) {
bp.Train(0,0,0);
bp.Train(0,1,1);
bp.Train(1,0,1);
bp.Train(1,1,0);
}
cout<< "0,0 = " << bp.Run(0,0) << endl;
cout<< "0,1 = " << bp.Run(0,1) << endl;
cout<< "1,0 = " << bp.Run(1,0) << endl;
cout<< "1,1 = " << bp.Run(1,1) << endl;
}
Nice post. Keep updating Artificial intelligence Online Trining
답글삭제